Introduction
Artificial intelligence (AI) has emerged as a revolutionary force in modern medicine, significantly reshaping diagnostics and treatment planning across various specialties [1,2]. In fields such as radiology and oncology, AI has had an unmistakable impact on improving diagnostic accuracy, enabling early disease detection, and optimizing treatment protocols [3,4]. For instance, in radiology, AI algorithms have revolutionized image analysis, facilitating more accurate interpretations and aiding in the early detection of illnesses [5].
The scope of AI integration ranges from diagnostics to patient management and care. Predictive analytics utilizing sophisticated machine learning (ML) algorithms are increasingly being employed to identify high-risk patients, predict complications, and personalize care plans [6]. This approach has ushered in a new era of proactive, patient-centric healthcare.
Moreover, AI is paving the way for precision medicine. By analyzing large datasets that include genetic profiles and patient histories, AI systems can provide treatments specifically tailored to the needs of individual patients. This approach significantly improves therapeutic effectiveness and minimizes side effects [7].
Despite these advances, general surgery lags significantly behind other medical fields in both AI research and clinical applications. The volume of medical articles published on the use of AI is markedly lower in the field of surgery, especially in general surgery (Fig. 1).
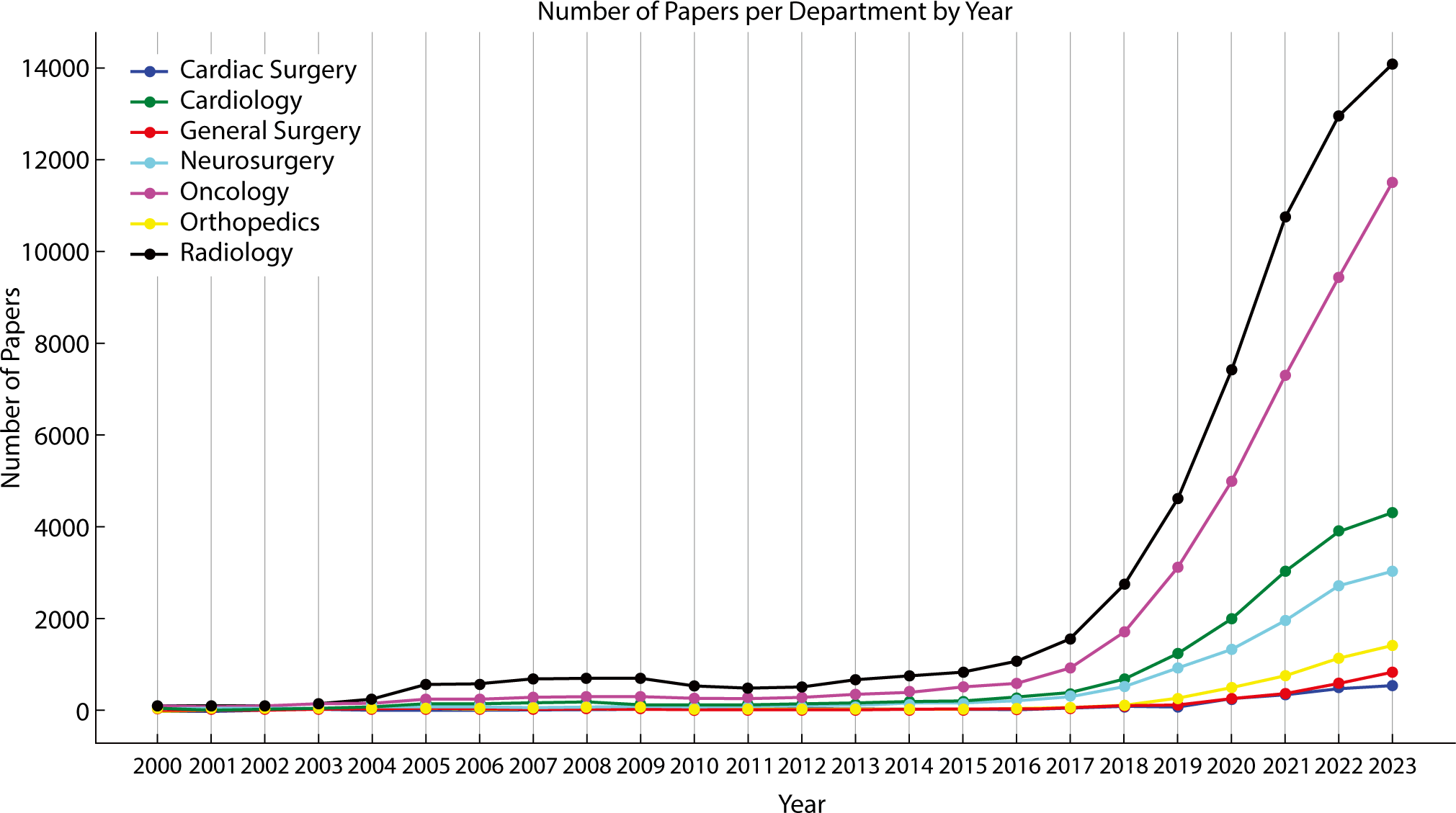
While specialized fields such as neurosurgery and cardiology are increasingly incorporating AI to improve surgical planning and robotic assistance, general surgery has been notably slower in adopting these advanced technologies [8,9].
The reasons for this delay are multifactorial. One of these reasons relates to the diversity and spontaneity of surgical procedures. General surgery is a dynamic field where some operations are predictable and can be scheduled in advance, while others are unpredictable and often rely on real-time decision-making in the operating room [10]. The variability in case types within general surgery complicates the collection of the extensive and consistent data necessary to train AI systems [10]. This issue is further exacerbated by the relative scarcity of focused research efforts aimed at integrating AI into general surgical workflows [10]. Therefore, locating studies on the use of AI in general surgery within databases like PubMed proves challenging.
This article summarizes the current state of research on the application of AI in medicine and explores the future direction of general surgery as it adapts to a rapidly changing medical environment. It includes a discussion on how AI can be integrated into various aspects of general surgery, ranging from preoperative analytics to postoperative care, as well as the steps required to overcome existing challenges.
Ethics statement
It is a literature database-based review; therefore, neither approval by the institutional review board nor obtainment of informed consent was required.
Pioneering artificial intelligence in the medical specialties of radiology, oncology, and cardiology
In modern medicine, the integration of AI has been particularly pronounced in specialties such as radiology, oncology, and cardiology. In radiology, AI algorithms have revolutionized diagnostic processes and enhanced the accuracy of image interpretation, which is crucial for early disease detection and treatment planning.
Recent studies on AI in radiology have produced important findings. Lång et al. compared the clinical safety of an AI-assisted screen reading protocol to that of the conventional double reading method used in mammography screening. The study involved 80,000 women and assessed early screening outcomes, including cancer detection rates, retest rates, false-positive rates, positive predictive values of the retests, and the types of cancers detected. In the intervention group, 244 tumors were detected, comprising 184 invasive tumors and 60 in situ tumors. Meanwhile, in the control group, 203 tumors were identified, with 165 being invasive and 38 in situ [11].
A randomized controlled trial conducted by Nam et al. demonstrated that AI-based, computer-aided design software enhances the detection rate of actionable lung nodules in chest radiographs of health-screening participants. The AI group exhibited a higher detection rate of actionable nodules compared to the non-AI group (0.59% vs. 0.25%). Additionally, the detection rate of malignant lung nodules was also higher in the AI group than in the non-AI group (0.15% vs. 0.0%). The rates of misdiagnosis and positive reporting were similar between the AI and non-AI groups [12].
Sachpekidis et al. have demonstrated that a deep learning (DL)-based tool for automatically assessing bone marrow metabolism in patients with multiple myeloma is feasible and correlates with clinically relevant disease parameters. There is a significant positive correlation between the visual analysis of PET/CT scans and the metabolic tumor volume (MTV) and total lesion glycolysis (TLG) values, following the application of all six 18F-fluorodeoxyglucose (FDG) uptake thresholds. Additionally, significant differences in MTV and TLG values were observed between patient groups across all applied thresholds.
The DL-based approach has demonstrated significant, moderate, positive correlations between bone marrow plasma cell infiltration and plasma β2-microglobulin levels, as well as with the automated quantitative PET/CT parameters, MTV and TLG [13].
Similarly, oncology has benefited from the use of AI, especially in the realm of personalized medicine. AI algorithms are employed to analyze patient data and predict responses to treatment, which allows oncologists to customize therapies based on the specific needs of individual patients. Clift et al. developed a clinically useful model that estimates the 10-year risk of breast cancer-related mortality for women at all stages of the disease. Additionally, they compared the outcomes of regression analyses with those of ML approaches. The final Cox model demonstrated good discriminatory power, evidenced by a Harrell’s C-index of 0.858 (95% CI, 0.853–0.864), and showed moderate calibration. The model's performance varied across ethnic groups, exhibiting the highest discriminatory power in Chinese women (Harrell’s C-index=0.931) and the lowest in Bangladeshi women (Harrell’s C-index=0.794). Moreover, the model generally performed well across various cancer stages, though its discriminatory power decreased as the cancer stage advanced [14].
Alaimo et al. have developed and validated a ML model to predict the early recurrence of intrahepatic cholangiocarcinoma following hepatectomy. The model, trained using 14 clinicopathological characteristics, demonstrates promising accuracy in predicting recurrences occurring within 12 months after surgery. It identifies tumor burden score as the most significant predictor of early recurrence, followed by perineural involvement. Additionally, the model's predictions of early recurrence strongly correlate with 3-year overall survival rates. Patients predicted to experience early recurrence exhibit significantly lower 3-year overall survival rates compared to those without such predictions [15]. A meta-analysis utilizing a substantial volume of recent data has been conducted to assess the effectiveness of AI in diagnosing lung cancer. The findings indicate that AI-assisted diagnostic systems achieve a sensitivity and specificity of 0.87, with a missed diagnosis rate and misdiagnosis rate each at 13%. The systems also show a positive likelihood ratio of 6.5, a negative likelihood ratio of 0.15, a diagnostic ratio of 43, and a combined sum of areas under the target operating characteristic curve of 0.93 [16].
Cardiology has kept pace with the AI revolution. AI systems in cardiology have been crucial in predicting cardiac events, thereby improving preventive cardiac care. A review has underscored the potential of AI for data interpretation and automated analysis in interventional cardiology procedures. ML techniques are employed in interventional cardiology for image reconstruction, interpretation, and analysis. ML models, including the lasso-penalized Cox proportional hazards regression model and the k-means clustering algorithm, have been utilized for predicting mortality and detecting the QRS complex, respectively.
ML algorithms have been developed for angiographic recognition, coronary angiographic interpretation, and intravascular ultrasonographic image segmentation. These algorithms have demonstrated promising outcomes in terms of recall, precision, accuracy, and agreement with expert analysts [17].
Another review has found that wearable devices, such as smartwatches and activity trackers, can collect and analyze long-term, continuous data on behavioral or physiological functions, providing healthcare providers with a more comprehensive picture of a patient's health compared to the traditional, sporadic measurements obtained through office consultations and hospitalizations. Wearable devices have numerous clinical applications, including screening for arrhythmias in high-risk populations and the remote management of chronic conditions like heart failure or peripheral artery disease [18].
Ishii et al. have developed and validated an ML-based model to predict future adverse events in patients with atrial fibrillation and stable coronary artery disease. Using randomized survival forest and Cox regression models, they created an integer-based risk score for all-cause mortality, myocardial infarction, stroke, and major bleeding, collectively defined as net adverse clinical events. This scoring system categorizes patients into three risk groups: low-risk (0–4 points), intermediate (5–8 points), and high-risk (≥9 points). The integer-based risk score has demonstrated strong performance in both the development and validation cohorts, exhibiting good discriminatory and calibration power. Decision curve analysis has shown a significant net benefit associated with this score [19].
The widespread adoption of AI in these specialties stands in stark contrast to its integration into general surgery, underscoring a significant gap in both research and clinical applications.
Advancements in artificial intelligence across various surgical departments
AI in neurosurgery has led to significant advancements in tumor identification and surgical planning. ML algorithms are employed to delineate tumors precisely, enhancing surgical accuracy and improving patient outcomes. Additionally, AI assists in predicting risks and developing personalized treatment plans.
Njiwa et al. investigated whether increased preoperative white matter (WM) 18F-FDG uptake can be used to predict surgical outcomes and compared the predictive performance of 11C-flumazenil (FMZ) and 18F-FDG–PET, using advanced ML techniques. They showed that at the group level, patients who were non-seizure-free (NSF) had more pronounced periventricular 11C-FMZ and 18F-FDG signal increases than patients who were seizure-free (SF). Five out of eight patients who were NSF had a periventricular WM signal increase in both 11C-FMZ and 18F-FDG, whereas only one out of eight patients who were SF had a periventricular WM signal increase in 11C-FMZ; and four out of eight had a periventricular WM signal increase in 18F-FDG, at the optimized threshold. Random forest classification correctly identified seven out of eight SF patients and seven out of eight NSF patients using 11C-FMZ images, but only four out of eight SF patients and six out of eight NSF patients, using 18F-FDG. The presence of ipsilateral medial temporal lobe hypometabolism predicted SF outcome status, while the absence thereof predicted NSF; nonetheless, 11C-FMZ-based methods performed better than 18F-FDG-based methods [20].
Ma et al. developed a noninvasive ML model to assist in identifying the grade and mutational status of molecular markers in intramedullary gliomas. This development is significant, as invasive biopsies for histopathological analyses carry a high risk of tissue damage. The results indicated that the Swin transformer-based model achieved high accuracy and dice similarity coefficients in the automatic segmentation of lesions during both the sagittal (SAG) and transverse (TRA) phases, with values of 0.9929 and 0.8697 for the SAG phase and 0.9978 and 0.8738 for the TRA phase. The neural network, based on the proposed multimodal fusion (SAG–TRA–clinical) features, demonstrated superior performance in predicting the grade and mutational status of molecular markers in intramedullary gliomas. The area under the receiver operating characteristic curve (AUC) was 0.8431 for grade prediction, 0.7622 for alpha thalassemia/mental retardation syndrome, X-linked (ATRX) status prediction, and 0.7954 for tumor protein p53 status prediction. The WHO-Mind model achieved the highest AUC, with a value of 0.8431 in the test task; both the WHO-Mind and ATRX-Mind models recorded the highest accuracy, each with a value of 0.8889 [21].
AI, particularly robot-assisted surgery, increases the precision of cardiac surgery. Liu et al. compared the clinical outcomes of robot-assisted cardiac surgery (RACS), utilizing the da Vinci robotic surgery system, with those of traditional open-heart surgery (TOHS). There were no statistically significant differences between the RACS and TOHS groups in terms of reoperation rates due to postoperative bleeding, mortality numbers, and treatment interruptions. The RACS group had shorter operative times and intensive care unit stays, fewer postoperative hospital days, and a quicker return to normal daily activities post-discharge than the TOHS group [22].
Fujita et al. compared minimally invasive direct mitral valve replacement via right thoracotomy with robotic mitral valve replacement to determine the feasibility of using robotic techniques for more complex lesions. They found that the mean complexity score for robotic repairs was significantly higher than that for thoracotomy. Additionally, the robotic group underwent a greater number of mitral valve replacements using polytetrafluoroethylene and performed fewer ablations. The overall cure rate was 100%, with no early mortalities or strokes observed in either group. In both groups, the mean postoperative residual mitral regurgitation was 0.3. The mean pressure gradient across the mitral valve was 2.4 mmHg in the robotic group and 2.7 mmHg in the thoracotomy group [23].
Another review article examined 27 studies that applied AI and big data to cardiac transplantation, categorizing them into four areas: etiology, diagnosis, prognosis, and treatment. AI-based algorithms demonstrated potential in predicting patterns and determining survival rates. However, the studies selected exhibited a significant risk of bias. The accuracy of AI-based models in predicting survival following cardiopulmonary transplantation and prognosis in thoracic organ transplantation was found to surpass that of traditional statistical methods. ML and DL techniques have improved diagnostic tools for detecting allograft rejection and predicting post-transplant survival. Additionally, ML has been employed to monitor the therapeutic levels of immunosuppressive drugs [24].
The role of AI in orthopedic surgery has been demonstrated in areas such as joint replacement and outcome prediction. AI is utilized for the customization of prosthetics and early diagnosis, thereby improving the success rates and effectiveness of orthopedic interventions.
Houserman et al. assessed the viability of an AI prediction model for knee arthroplasty, utilizing three-view radiography to determine if patients with knee pain required total knee arthroplasty (TKA), unicompartmental knee arthroplasty (UKA), or no arthroplasty at all. The AI model achieved an accuracy of 87.8% and a quadratic-weighted Cohen's kappa score of 0.811 in the holdout test set. It performed exceptionally well in determining whether a patient was a candidate for surgery, reaching an accuracy of 93.8%. The multiclass AUC scores for the three categories—TKA, UKA, and no surgery—were all above 0.95, specifically 0.974, 0.957, and 0.98, respectively. The AI/ML model, as well as AI models in general, demonstrated potential in predicting whether patients are suitable candidates for UKA, TKA, or no surgery [25].
Jang et al. utilized DL to automate the measurement of leg length discrepancy (LLD) using pelvic radiographs and to compare the LLD based on different anatomical landmarks. The DL algorithm has successfully measured LLD on pelvic radiographs by utilizing various combinations of landmarks, achieving intraclass correlation coefficients (ICCs) ranging from 0.73 to 0.98. Measurements of LLD using the teardrop and greater trochanter landmarks have shown an acceptable level of agreement, with an ICC of 0.72 [26].
Advancing artificial intelligence in general surgery: current research landscape and future directions
Research on AI in general surgery is expanding into numerous areas, reflecting the diverse applications of AI in this multifaceted field. The integration of AI into laparoscopic surgery enhances visualization, accuracy, and decision-making during procedures.
In robotic surgery, AI has been leveraged to improve the precision and autonomy of robotic systems, marking a significant shift toward more advanced surgical techniques.
Endo et al. discussed the impact of an AI system on identifying anatomical landmarks associated with reduced bile duct injury during laparoscopic cholecystectomy. After viewing a 20-second video where the AI highlighted landmarks, 26.9% of the images were annotated differently, primarily along the gallbladder line of the extrahepatic bile and cystic ducts. Of these changes, 70% were considered safe. The AI system assisted both novices and experts in identifying landmarks such as the Rouviere sulcus and the inferior border of the liver, S4. It encouraged changing perspectives in 70% of cases, in a way that was considered safe [27].
Zhang et al. explored the feasibility of conditional autonomy in robotic surgery, specifically focusing on robotic appendectomy. This approach involved using demonstration data gathered from a human operator performing appendectomies in a simulated robotic environment to teach the system the movements and trajectories of the robotic instruments. Extensive validation in a simulated environment, utilizing the da Vinci research kit, demonstrated that the proposed method can perform appendectomies semi-automatically. A framework based on this method could decrease the total working path length, completion time, and appendix stump length, while preserving a high similarity to the demonstrated trajectories [28].
In addition, AI models for surgical risk assessment are currently being developed. These models use patient data and preoperative indicators to predict postoperative complications, aiming to tailor surgical approaches to the specific risks of individual patients. Additionally, AI plays a crucial role in surgical planning, especially in complex procedures. Here, AI-driven image interpretation aids surgeons in making informed decisions.
El Moheb et al. demonstrated that the AI risk calculator, Predictive OpTimal Trees in Emergency Surgery Risk (POTTER), surpassed the surgeon's gestalt in predicting postoperative mortality and outcomes for patients undergoing emergency surgery, except in cases of septic shock. Risk prediction for mortality, bleeding, and pneumonia improved when surgeons used POTTER, although there was no significant improvement for septic shock or ventilator dependence. The AUC was calculated to evaluate the predictive performance of surgeons who used POTTER compared to those who did not [29].
The postoperative phase has also benefited from AI, particularly in the areas of wound analysis and care. AI applications here concentrate on analyzing images of wounds and predicting healing outcomes, potentially leading to more personalized and effective postoperative care strategies.
Tomé et al. highlighted the necessity of AI by demonstrating the challenges in predicting postoperative infections using only correlated data. According to their research, postoperative infections occurred in 24 out of 349 operations, which accounts for 6.89% of all surgeries in their database. Correlation tests employing Pearson and Spearman coefficients indicated a weak correlation between the risk factors and the incidence of infection. An artificial neural network designed for pattern recognition successfully predicted infections in 77.3% of cases, achieving an AUC of 0.9050. Among the misclassifications, seven cases were incorrectly identified as having an infection when none was present, representing 2.0% of the data. Conversely, five cases were incorrectly identified as not having an infection when one was present, representing 1.4% of the data [30].
Overall, these diverse areas of AI application in general surgery underscore the potential of AI to transform various aspects of surgical practice, from preoperative planning to postoperative care [31,32]. As research progresses, the role of AI in general surgery is anticipated to grow, setting the stage for more innovative and effective surgical practices.
Bridging the artificial intelligence gap in general surgery
The integration of AI into specialties like radiology and cardiology has significantly improved diagnostic accuracy and patient care. This stands in stark contrast to its use in general surgery. The disparity underscores the unique challenges faced in general surgery, which include the variability of surgical procedures and the difficulty in capturing comprehensive datasets for AI training.
Understanding the challenges and successful strategies used in other specialties can provide valuable insights for adapting AI applications in general surgery, suggesting a more focused approach to research and development in this area. The primary issue is the relative scarcity of research directed toward implementing AI in general surgical environments. The inherent variability and complexity of general surgical procedures pose significant challenges in standardizing AI applications, which in turn complicates the integration of AI. Additionally, constructing comprehensive and uniform datasets, crucial for training AI, continues to be a major hurdle in this field [33].
Despite these challenges, there are significant opportunities in general surgery where AI can make substantial contributions, such as in risk assessment and surgical planning [34–36]. Success stories from other medical and surgical fields offer a blueprint and valuable insights for integrating AI into general surgery. By drawing on these experiences, general surgery can tailor AI tools to meet its unique needs, potentially transforming patient care and surgical outcomes [36–38].
Promoting research on AI and the application of AI in general surgery requires fostering interdisciplinary collaboration across various fields, establishing standardized data collection and sharing protocols, securing dedicated funding, and integrating AI education into medical training. It is necessary to address ethical considerations and provide regulatory support to build trust in AI applications. Pilot projects and clinical trials are essential to demonstrate the efficacy and safety of AI technologies in clinical settings, paving the way for their integration into general surgery to enhance outcomes and patient care.
Conclusion
The future of AI in general surgery is poised for transformative growth, driven by emerging technologies. Surgical robotics are increasing precision and safety, virtual reality simulations are providing unparalleled training experiences, and predictive analytics are improving postoperative care.
Focusing research on these areas could significantly advance the field of general surgery, aligning it with the successes observed in other medical fields and opening new avenues for enhancing patient care. In radiology, oncology, and cardiology, AI has already begun to transform patient care by improving diagnostic accuracy, providing predictive analytics, and facilitating personalized treatment plans.
However, the field of general surgery stands at the threshold of a significant technological evolution, facing unique challenges that hinder the integration of AI. To effectively incorporate AI into general surgery and address delays in current research and development, interdisciplinary collaboration is essential. This requires forming partnerships among medical practitioners, AI technologists, data scientists, and policymakers. These collaborative efforts are vital for managing the complexities of general surgical procedures, standardizing AI applications, and constructing the comprehensive datasets required for AI training.
By leveraging diverse expertise, AI tools can be tailored to meet the unique requirements of general surgery, thereby improving surgical outcomes, procedural efficiency, and patient care.
The path forward requires a concerted effort to bridge this gap, focusing on the development of AI tools tailored to the specific needs of general surgery, from preoperative planning to postoperative care. Embracing AI in general surgery not only promises to improve surgical outcomes and efficiency but also represents a critical step toward a future where healthcare fully leverages technology, marking a new chapter in the quest for enhanced patient care.